The imperative of robust data governance in the age of AI
Data governance plays a crucial role in today's AI-driven world. From understanding the potential risks of poor data oversight and the implications of new AI tools to ensuring data security and quality, learn how stringent data governance can pave the way to success in the age of AI.
2024-09-10
Annette Memishofski, Managing Director, Data Insights Practice
The notion of AI ruling our lives represents a common fear. Who determines the route you will take? Who is helming the initiative? How will you know you're staying on course? But it's not like this is uncharted territory. During the last 30 years, data access has gone through a remarkable transformation, starting from simple paper records stored in basements to sophisticated AI-driven data ecosystems.
However, in a world increasingly driven by data, the real peril isn't AI itself, but rather how we govern our data. With poor data governance, the waters are murky and dangers lie in wait just beneath the surface. Organizations that master data governance will sail more smoothly to AI success.
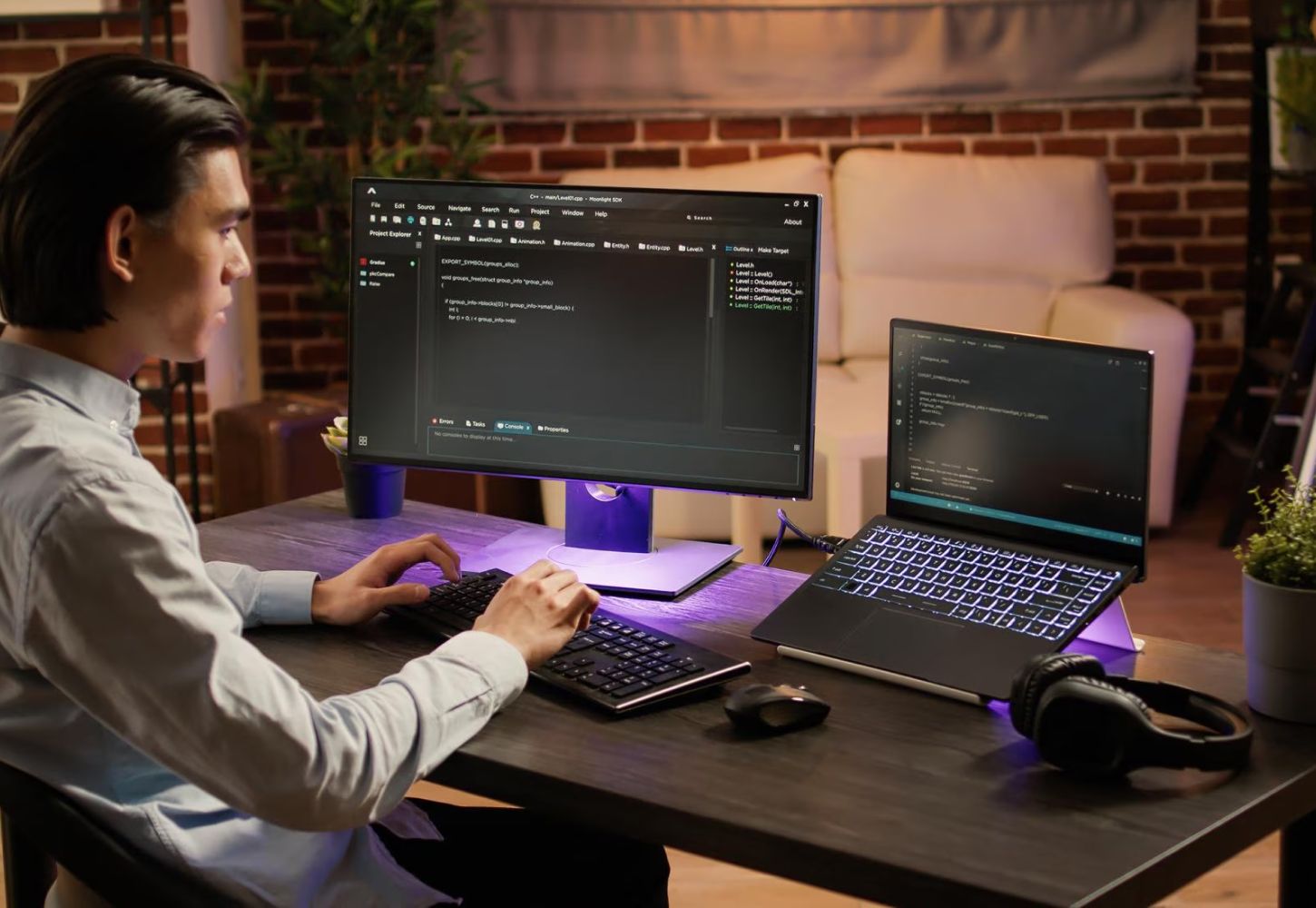
Unfurl the sails, with caution.
AI’s potential is truly revolutionary. AI lets you search through all of your company’s hard drives, millions of emails, PDFs, spreadsheets, logs and all sorts of other essential business documents. It can then give you, in seconds, critical summaries, recommendations or even business decisions that drive your operations. We are now in the midst of a transition from knowledge-based, generative AI-powered tools—such as chatbots that answer questions—to generative AI-enabled agents that perform complex, multistep workflows. In essence, the technology is evolving from mere thought to actionable execution.
Tempering the excitement, however, CIOs and CTOs are now starting to question some of the implications of these incredible new tools. They’re asking: How do you govern that? How do you make sure the information you put into your AI engine is of a high enough quality that the AI system provides your users with quality answers? Are we giving away trade secrets by providing access to this data? And can we even trust the answers AI gives us?
And that leads to other critical questions: How do you secure your data? How do you make sure the right people are getting access to the right data? When someone makes a query, they need assurance that they’re getting good information in response. This is true whether it’s an internal query from one of your business units, or perhaps you’re monetizing some of your data and opening yourself up to external queries.
I have worked in data for a long time, so I can tell you that while the AI movement is new, it’s not as though we haven’t seen new technologies come on board before. We will get through this and I will tell you how — but first, a history lesson.
The early days of data storage
Data access began with the digitization of paper documents into microfiche. This made data more accessible but also required strict governance to ensure only public information was available to the public. Private information remained restricted to authorized leaders, emphasizing early forms of data governance.
The client-server era
With the transition to client-server applications, data spread across desktops and laptops, necessitating tighter access and governance controls. Data was dispersed across multiple machines within organizations, requiring new strategies to manage and secure it effectively.
The internet revolution
With the advent of the Internet, companies began publishing static pages, providing the public with more detailed information about company performance, products and annual reports. Dynamic content soon followed, allowing personalized access to transaction-level data, such as orders and inventory, and interactive dashboards. This shift further emphasized the need for robust data governance and access controls.
The challenges of data quality
As data access expanded, so did challenges related to data quality and consistency. Different departments relied on dashboard information to make business decisions, highlighting inconsistencies and necessitating various data controls. These controls primarily focused on structured data stored in relational databases and flat files.
The rise of AI
Throughout this journey, the common theme has been that increased data accessibility requires stronger governance and tighter controls.
Today, as we embrace AI initiatives across enterprises, the data landscape has changed yet again. AI technologies leverage not only structured data but also unstructured data from emails, Google Drives, SharePoint lists and various document formats stored in many different places. While AI enhances competitiveness and productivity, it also makes robust data governance more important than ever.
The evolution of data access over the past few decades underscores the critical need for comprehensive data governance and stringent access controls. As we move forward, embracing new technologies like AI, organizations must prioritize governance to ensure data integrity, quality and security at every stage.
Working with the tides of AI
Data integrity and data security
When we’re talking about data governance in the age of AI, not everything can be used for AI. For instance, you might have contracts with clients that restrict how you store information. The contracts themselves contain a lot of useful information, too. But again, they are typically confidential. So you need to have a plan for a hybrid environment, with some things in the cloud and others on premise, and documentation that details how data is stored and used.
With other data you want to incorporate into AI, such as emails, you will need to anonymize it or aggregate it so you can still monetize it externally, or feed it to divisions and departments within the organization without having to give up the details.
This is not a completely different problem from those we have faced before, but it is a lot easier to control a structured relational database than it is to parse unstructured data by going through emails, contract files and PDFs stored on hard drives, Google Drives or SharePoint sites.
What not to do – but what I have seen some do
I know of one company that is so fearful of AI and how it might compromise its data that it banned employees from using ChatGPT. That’s exactly the wrong approach.
An HR employee at this company had to rewrite 200 job descriptions, just the kind of tedious, time-intensive, repetitive work that AI can do much more efficiently. She went to ChatGPT and started producing well-worded, accurate descriptions. But when her boss found out, instead of rewarding her innovative thinking, they shut the project down. Rather than avoiding all AI tools, when leveraging external AI tools, you should consider privatized versions to gain more control over data and ensure the data is used appropriately.
Then again, other companies are veering to the other side, saying, “Hey, let's just open it up to everybody,” without any thoughts about what they are putting out there. You need to keep your data secure, and anonymize it when necessary. You can make the most of AI in the day to day, while ensuring your and your customers’ data security.
What you need to do – and what I’m not seeing most people do
Implementing data governance in AI-powered enterprises requires a strategic approach. Here are some steps to guide the process:
1. Assess your organization's current data practices.
Identify gaps and areas for improvement in data management, quality and security. Continually refine governance policies and processes to address the evolving nature of AI technologies. Make processes lightweight and sustainable.
2. Develop a comprehensive data governance framework that outlines policies, standards and processes.
Ensure the framework aligns with your organization's goals and regulatory requirements. It serves as a blueprint for implementing data governance across the organization, ensuring consistency and compliance.
3. Develop a two- to three-year roadmap and implementation plan for AI.
Given how fast AI is evolving, a five- or ten-year roadmap is an exercise in futility. Even a two-year plan might be too long. Consider starting with small successes within individual business units.
4. Appoint data stewards to oversee data governance efforts.
Data stewards are responsible for managing and overseeing data assets. They ensure data quality, integrity and security by implementing governance policies and procedures. Ensure they have the necessary authority and resources to implement and enforce governance policies. Set up validation processes so executives and others can trust insights and reports generated through AI. Consider stamp-of-approval programs.
5. Implement data cataloging to create an inventory of data assets.
This enhances data accessibility and promotes better decision-making. Use advanced tools and technologies to automate the cataloging process and enhance data accessibility.
6. Train employees on how to effectively leverage AI.
Employees must be properly educated about AI and understand what their new roles may involve before they can harness AI to increase productivity. Also, plan for potential workforce impacts.
You will find it easier to implement these items if you work with expert partners who understand both the technical and business impacts of AI to help navigate challenges and ensure strategic alignment. Your partner should have a proven track record in not only data warehousing and data security, but also understand a number of the technologies that are out there and the ways you might use open-source technologies. They should be able to bridge that gap from your current data platform to get you to the next level.
You don’t have to rip out your old system. You can stay with your current platform and layer new tools on top of it, along with critical security measures. You can manage the move to AI and still keep your data secure. You can employ a data and AI strategy now and still keep up-to-date as technologies advance.
By implementing robust data governance frameworks in your AI initiatives, organizations can ensure data integrity, quality and security. As we move forward, the importance of data governance will only continue to grow, making it a critical focus for enterprises worldwide.
It will take some work, but if you’re thoughtful and you start now, you will find yourself on a path to unlocking greater efficiency and productivity through AI.