Detecting and Solving for Fraud
Fraud poses a significant challenge across various industries, leading to substantial financial losses and reputational damage. As technology advances, fraudsters continue to refine their techniques, making detection and prevention more complicated. In this evolving landscape, innovative approaches using Language Models (LLMs) and Vector Databases have emerged as powerful tools to combat fraud with greater accuracy and efficiency.
Can you detect fraud? In time? Or do you even know it's happening until it's too late? Fraud continues to be a significant challenge affecting a wide range of industries, leading to considerable financial losses and damaging reputational risks. As technology evolves, so too do the sophisticated techniques used by fraudsters, making detection and prevention increasingly complex.
In response, cutting-edge strategies utilizing Language Models (LLMs) and Vector Databases have emerged as formidable tools in the battle against fraud. These innovative solutions enhance the ability to identify, analyze and mitigate fraudulent activities, offering unprecedented accuracy and efficiency in safeguarding businesses and maintaining trust.
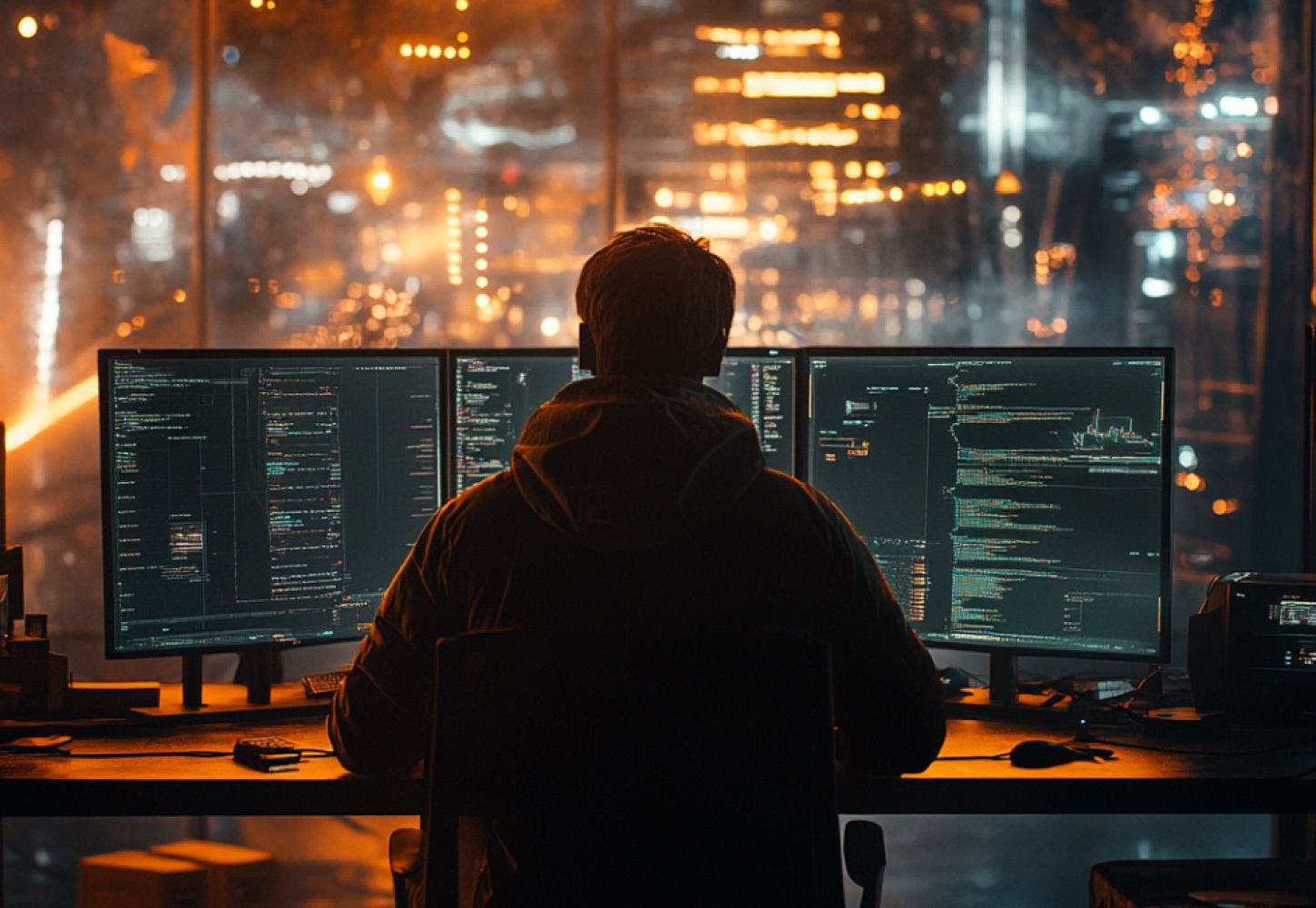
Understanding Fraud Detection
Fraudulent activities frequently leave behind traces, hidden within intricate and seemingly unrelated patterns of data. Traditional fraud detection systems have typically depended on rule-based approaches or statistical models. While these methods have their merits, they often fall short when it comes to adapting to the rapidly evolving tactics used by fraudsters, who employ increasingly complex and disguised schemes.
In today's digital landscape, where cunning deception abounds, it's crucial to move beyond conventional systems and embrace more adaptive, intelligent solutions capable of uncovering even the most subtle indications of fraud.
Leveraging Language Models (LLMs)
Language Models, such as OpenAI's GPT (Generative Pre-trained Transformer) series, have revolutionized data analysis by comprehending and processing unstructured data like text. When applied to fraud detection, LLMs excel at analyzing textual data—such as claims descriptions, transaction notes or communication records—extracting nuanced insights and identifying anomalous patterns that evade conventional methods.
By training Language Models (LLMs) on extensive datasets of historical fraud cases, these advanced models become adept at discerning subtle linguistic cues, identifying associations and detecting anomalies within vast amounts of data. They are capable of uncovering irregularities and spotting unusual language patterns in claims or communications, effectively flagging them for further scrutiny. Beyond this, the powerful contextual understanding capabilities of LLMs allow them to recognize and analyze emerging fraud schemes, even when such schemes lack recognizable patterns or specific keywords. This adaptability ensures that organizations are equipped to proactively identify and address sophisticated and novel fraudulent activities, significantly enhancing their defense mechanisms.
Utilizing Vector Databases for Pattern Matching
Vector databases, particularly those powered by advanced algorithms like cosine similarity or neural network-based embeddings, offer a sophisticated means of storing and comparing complex patterns or representations derived from the LLMs.
Once the LLM identifies potential fraudulent patterns within the data, these patterns are converted into numerical vectors and stored in a vector database. These databases then leverage similarity metrics to match these vectors with known fraud patterns or clusters within the database. This enables quick and accurate identification of potential matches between new instances and historical fraud cases.
The Synergy: LLMs and Vector Databases
The integration of LLMs with vector databases forms a potent synergy. LLMs excel at understanding complex data patterns, while vector databases provide an efficient structure for storing and matching these patterns against historical fraud cases. This powerful combination significantly boosts fraud detection capabilities by:
- Identifying sophisticated fraud schemes that often slip through conventional rule-based systems due to their intricacy and subtlety.
- Adapting to emerging fraud tactics and evolving patterns through continuous learning, ensuring preparedness against ever-changing fraudulent behaviors.
- Accelerating the detection process by swiftly matching current data patterns with past fraud instances, enabling real-time responses to potential threats.
Together, LLMs and vector databases provide a dynamic and robust approach to safeguarding organizations from the increasingly complex landscape of fraudulent activities.
Conclusion
In the ever-evolving landscape of fraud, the application of Language Models in tandem with Vector Databases represents a pivotal advancement in fraud detection capabilities. By harnessing the power of artificial intelligence and advanced data storage techniques, organizations can bolster their defenses against fraudulent activities, minimize financial losses and protect their reputation.
The continuous evolution of these technologies promises even greater accuracy and efficiency in detecting and mitigating fraudulent behavior, ensuring a more secure environment across industries.
If you're interested in discovering more about Ollion's approach to fraud detection, reach out to us for more information. Together, let’s review and enhance your security posture.